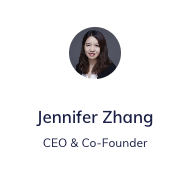
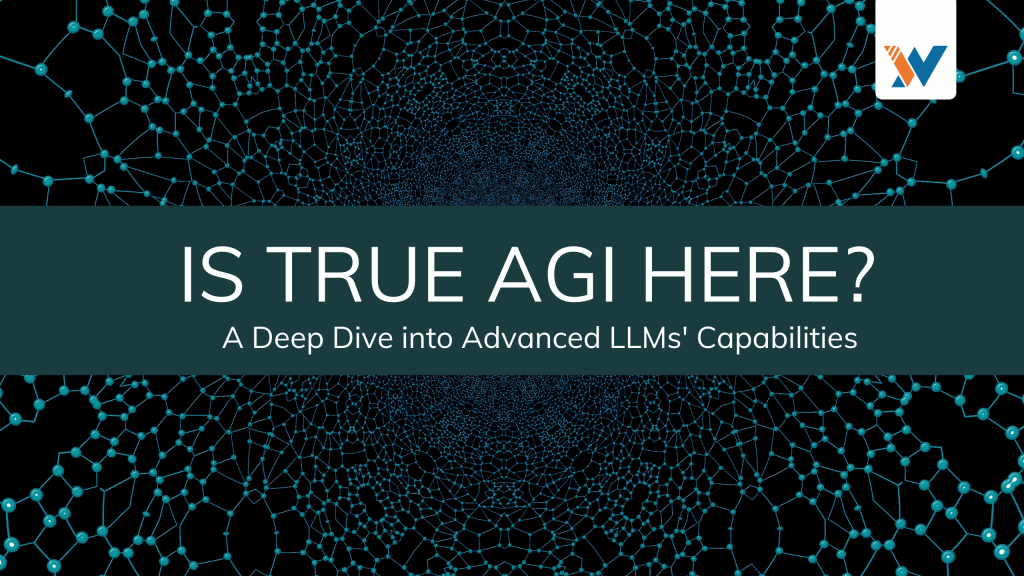
On Oct 10th, two renowned AI researchers from Google and Stanford published an intriguing essay, claiming Artificial General Intelligence (AGI) is already upon us. Shortly thereafter, Open AI quietly changed its core value to be an AGI focused company. These recent developments may signify a pivotal moment, marking our entrance into the AGI era. Today, we will delve into the trending topic of AGI, exploring its definition, capabilities, and share our insights into this fascinating sphere of true AGI.
From Narrow AI to AGI
In the “Artificial General Intelligence is Already Here” essay, the two writers consider current frontier AI large language models (LLMs) such as ChatGPT, Brand, LIama and Claude to be true examples of AGI. Because these LLMs have already demonstrated advanced capabilities beyond their predecessors – the so-called narrow AI.
Narrow AI, or weak AI, typically perform a single or predetermined set of tasks, and it is explicitly trained for these tasks. While these narrow AI models can complete tasks efficiently, they can not extend beyond pre-determined scope and workflow curated by humans. Voicebot is an example of narrow AI technologies. It is capable of driving impressive business results via round-the-clock services and automation, but can only stick to rigid pre-determined dialogue flows.
Contrarily, AGI, also known as strong AI or full AI, can perform a broad spectrum of tasks, most importantly, including novel tasks that transcend the limits set by their training or their creators. Theoretically speaking, LLM’s performance is based on the amount of training data. LLMs will achieve the goal assigned to them, and their performance will improve with the increase of input data. However, LLMs performance actually exceeds scientists’ expectations by demonstrating that they can perform new tasks far beyond their training.
An example demonstrating such surprising capabilities was showcased during an LLM performance test last year. There was one prompt asking LLMs to guess the movie name from the emoji below:
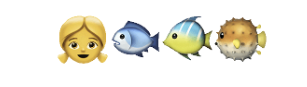
The LLMs with the most simple training dataset produced a completely unreasonable guess: “The movie is a movie about a man who is a man who is a man.” Medium-complexity models had a better but still wrong guess: The Emoji Movie. But the model with the most complex training dataset guessed correctly in a single try: Finding Nemo. “Despite trying to expect surprises, I’m surprised at the things these models can do,” are the remarks made by a computer scientist involved in the test.
Understanding the abilities of AGI
AI experts consider today’s trailblazing AI models to have achieved several facets of AGI. Let’s examine these areas through use cases and experience the intelligence level of present-day advanced AI systems and the tasks they can accomplish.
Contextual learning: a key aspect of AGI
The emoji guessing example above demonstrates remarkable contextual learning abilities of advanced LLMs. This feature, deemed crucial by many AI experts, has allowed sophisticated LLMs to undertake tasks that surpass their training data. Instead, such LLMs can handle new tasks based on any directive that can be described in prompts.
NeogenixAI’s experts recently built a prototype of a meta prompt system. Using our meta prompt system, users no longer need to manually create prompts for LLMs to perform tasks. They simply provide an example of an input-output pair, and LLMs can swiftly self-generate a prompt capable of managing similar tasks within minutes, as demonstrated with a new math calculation in the video below.
https://www.youtube.com/watch?v=eNFUq2AjKCkAutonomous decision making and tool use
The above self-prompting example leads us to another key trait of AGI – decision making. The decision making ability enables LLMs to plan for task execution and call other tools or agents to take action. This forms an LLM powered autonomous agent system, also known as LLM agent. Equipped with agents or tools, LLMs are like brains with limbs, ready to tackle practical challenges beyond conversation abilities.
Picture an LLM agent helping change a client’s flight booking. It must first decide what information is needed, like the airline’s change policy and alternative flight options. It can then utilize tools like documentation APIs and flight databases to gather the necessary details to assist the client.
Multimodality: another leap into AGI
The recent unveiling of GPT-4V signifies the rise of large multimodal models (LMMs). LMMs enhance LLMs by integrating multi-sensory abilities, leading to a more robust general intelligence. While previous multimodal models could operate on diverse modalities and generate varied formats, such as texts, images, videos, and audios, they could only comprehend text-only prompts. LMMs, conversely, surpass text-based prompts, aligning more closely with human natural intelligence.
GPT-4V can “see” the world with image prompts
As an LMM, GPT-4V can interpret the world using image prompts. Microsoft’s report has disclosed some GPT-4V’s remarkable multimodal intelligence. It can identify social settings in images such as a waitress serving clients, count numbers of objects and people, recognize celebrities, and generate descriptive captions. Additionally, GPT-4V can comprehend video content and provide content summary, understand frame sequences and even predict the following frame.
Multilingual understanding
Languages are considered as a crucial aspect of general intelligence. LLMs can communicate in multiple languages and translate between them, even for language pairs without example translations in the training data. Additionally, they can “translate” between natural languages and computer programming languages.
With the latest LMMs like GPT-4V, they can recognize text overlays on images, and use the acquired information to complete the language translation task requested. Furthermore, they are able to recognize social norms in different cultures, such as weddings organized in traditional styles from Japan, China and India.
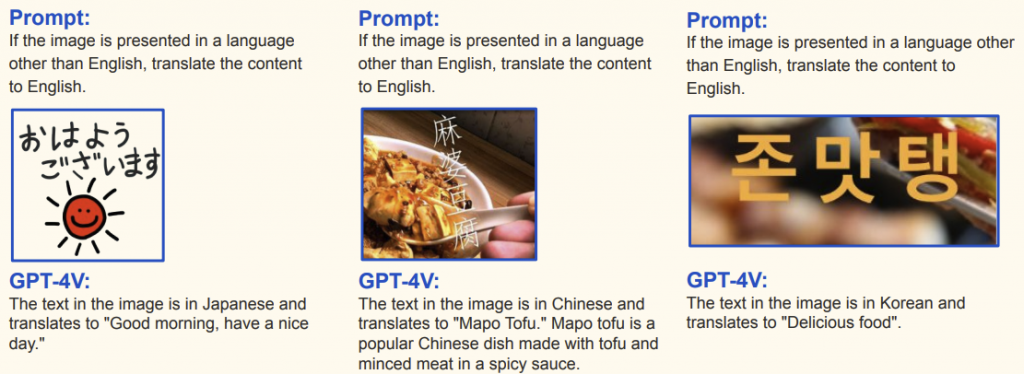
Exploring true AGI: addressing skepticism
Criticisms of true AGI
While leading LLMs and LMMs exhibit potent capabilities, the debate on whether we’ve attained true artificial general intelligence remains.
Noam Chomsky, father of modern linguistics argues that LLMs are purely based on statistical probabilities, which is far away from complex human intelligence relying on reason and language use. This limits their flexibility compared to complex human cognition.
Similarly, linguist Emily M. Bender compares large language models with “stochastic parrots.” She asserts that LLMs do not truly understand the real world, but simply calculate the statistical probability of words appearing. Then, like parrots, they randomly generate seemingly reasonable phrases and sentences.
Famous AI critic Gary Marcus also aligns with these criticisms. He further highlights the persistent issue of AI hallucinations, which make these AI models unreliable. Additionally, Marcus mentions lack of transparency from AI giants like OpenAI and Microsoft. He claims that the broader scientific community can not validate their claims of AGI, as there is no access to the underlying mechanisms or training data.
Our perspectives on true AGI
LLMs and real-world comprehension: beyond statistical probabilities
It’s true that LLMs are created based on statistical probabilities. But there is evidence suggesting they do exhibit real-world comprehension.
One such evidence is the Othello-GPT study by Harvard and MIT researchers. The study involved training a GPT language model on the board game Othello. During training, the LLM saw only move sequences without explicit knowledge of the game’s 8×8 square board or rules. After training, the GPT model could competently play the game. This demonstrates that it is not merely stochastic parrots, but possesses a genuine understanding of the board position, game rules and legal moves. Renowned AI scientist and entrepreneur Andrew Ng asserted in response to this study that LLMs do, to some extent, comprehend the world.
A more recent MIT report further proved this viewpoint. The new research revealed LIama-2’s ability to understand fundamental dimensions like space and time, suggesting LLMs can internalize physical world understanding.
LLMs’s self-learning ability can mitigate hallucinations
We also acknowledge that current advanced LLMs exhibit flaws, with hallucinations being a significant issue. However, when possessing contextual learning abilities, these LLMs can continue to self-evolve and self-learn from their own experience. We’ve already seen such remarkable breakthroughs. One such example is GPT-4V’s ability to identify counterfactual details in images, even when deliberately misguided in prompts.
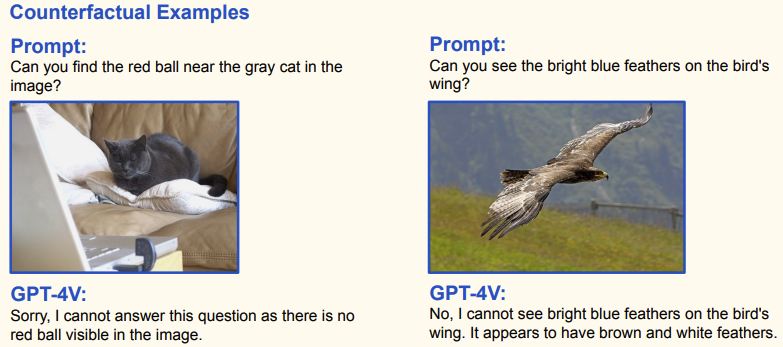
AI transparency and the dawn of AGI
Regarding the criticism about leading AI companies’ lack of transparency on their models’ inner workings and training data, we concur with the observation. But we don’t agree to the conclusion that it renders any AGI claims impossible to verify.
The AI landscape is not monopolized by one or two dominant players, leaving the rest of the world in the dark. Instead, the current AI community comprises a myriad of players, from tech behemoths to innovative startups, and advanced open-source LLMs like LIama-2 are readily available to the general public. The current level of openness, transparency, and diversity of perspectives surpasses any previous era.
With a host of cutting-edge AI large language models, such as ChatGPT, Brand, LIaMA and Claude, all displaying signs of general intelligence, we believe we’re embarking on the dawn of a true AGI era.